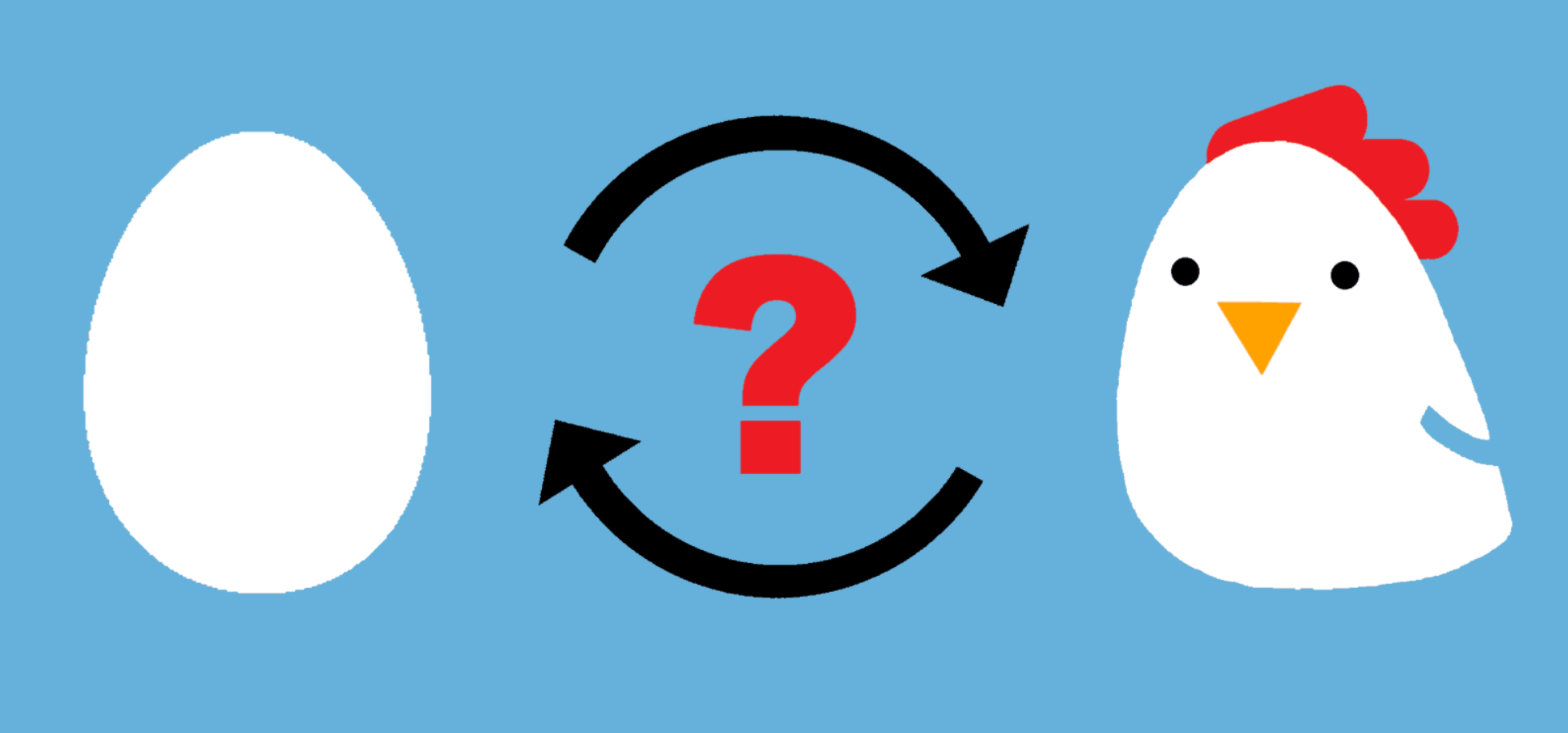
What is causal Inference?
Causal Inference is the process of drawing conclusions about a causal connection. The question that is asked most often for research in health, social, and behavioral science is about causal nature. Causality helps us understanding the Cause & Effect relationship in the data.
Why Causal Inference in important?
Understanding the cause and effect is the natural way of making an inference, the closest one to the real-world model. To build a strong AI we need to emulate how humans think about this cause and effect relationship. Knowing and asking "why" about the data is important, Causality can help us answer this question.
Abstract
Causal Inference is all about figuring out the Cause & Effect relationship between the features. The critical intellectual distinction between humans and machines is the ability to define goals and reason towards achieving them. Causality can help us answer some very important questions. Research under causal Inference will involve exploring topics like causal reasoning, methods for causal inference etc. The meeting will be mainly focused on discussion and brainstorming ideas about research papers in the field, implementation methods and coming up with new project ideas in the field. Considering this is a new field, there are a lot of research opportunities where members can even get their ideas published.
Resources
Checkout the video for more information.Read more about it on eBook
Research Head
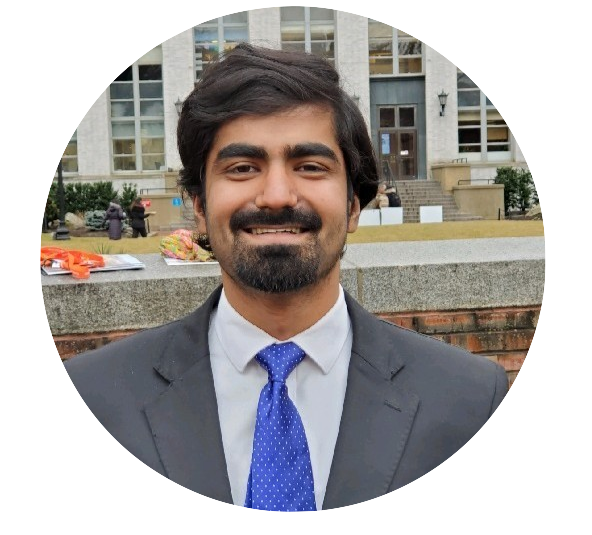